-
Practical Guide to Interactive Experience Techniques for 2024: Discover Proven Approaches
Interactive Experience Techniques: A Comprehensive Guide In today’s rapidly evolving digital landscape, creating engaging and memorable user experiences has become a necessity for brands and businesses. One of the most effective strategies to enhance user interaction is through interactive experience techniques. According to recent studies, users are more likely to engage with content that is…
Latest Posts
-
Practical Guide to Interactive Experience Techniques for 2024: Discover Proven Approaches
Interactive Experience Techniques: A Comprehensive Guide In today’s rapidly evolving digital…
BY
-
Odkryj 7 Sekretnych Zasad Sezonowych Konkursów z Nagrodami
Seasonal Contests with Prizes Seasonal contests have become an exciting way…
BY
-
Rabat: 5 Najlepszych Sposobów na Większe Zyski Teraz
Rabat Najlepsze Zyskaj Teraz Rabat, the capital city of Morocco, is…
BY
-
Entdecken Sie die besten Rabatte auf ausgewählte Angebote im Jahr 2024!
Rabatte auf ausgewählte Angebote: Maximieren Sie Ihre Einsparungen Wussten Sie, dass…
BY
-
4 Эффективные Способы Получения Бесплатных Пробок в 2025 Году: Узнайте Как!
Trendy na bezpłatne próbki In an era where consumers are increasingly…
BY
-
Practical Ways to Receive Free Samples in 2024 – Discover the Best Options!
Jetzt kostenlose Proben erhalten In einer Welt, die ständig im Wandel…
BY
NATURE
ADVENTURE
TRAVEL
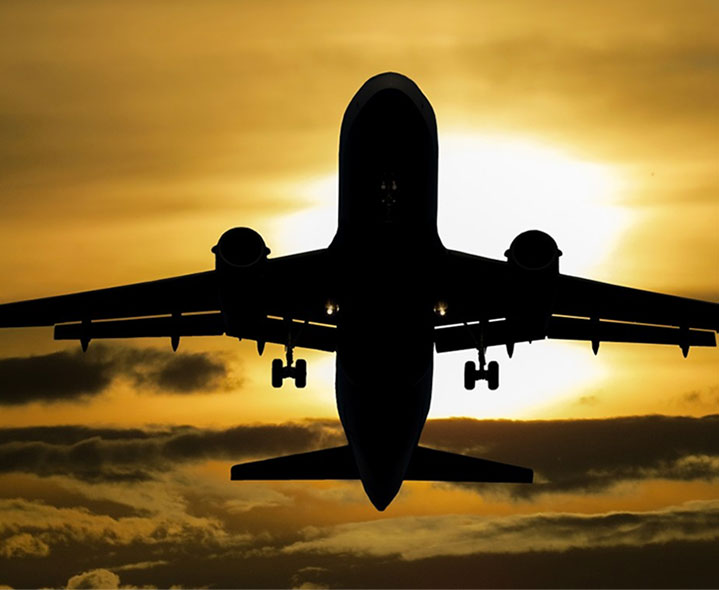
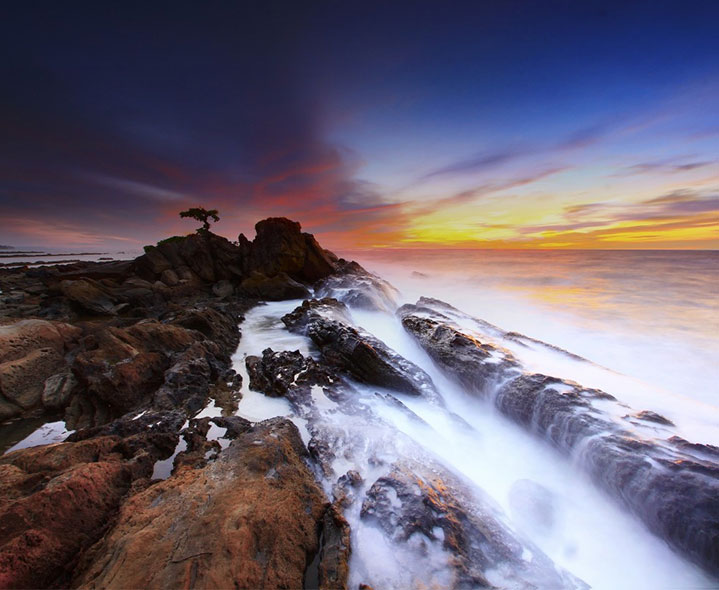
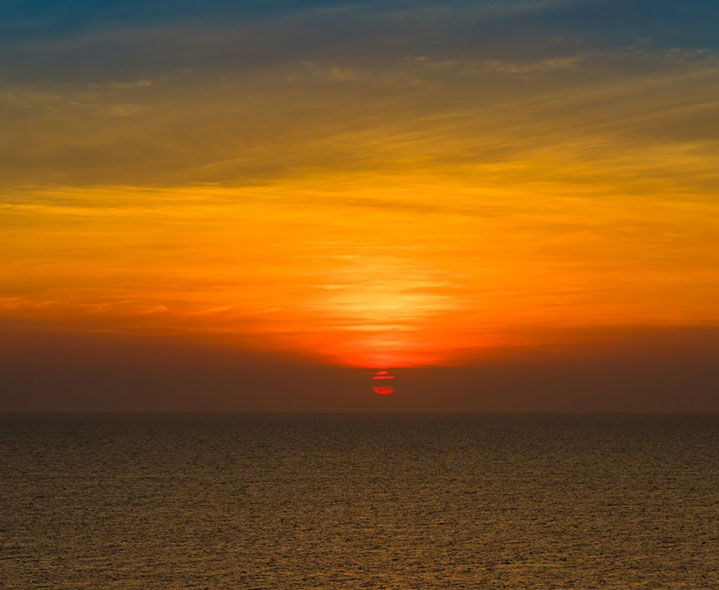
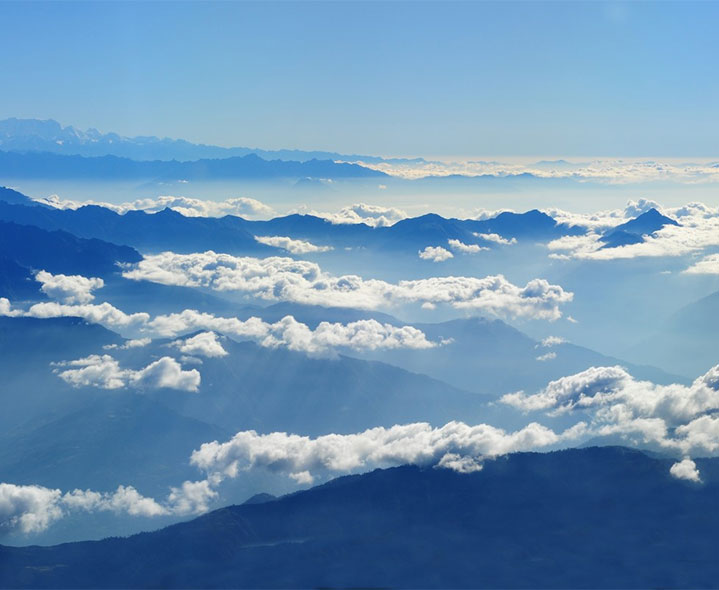
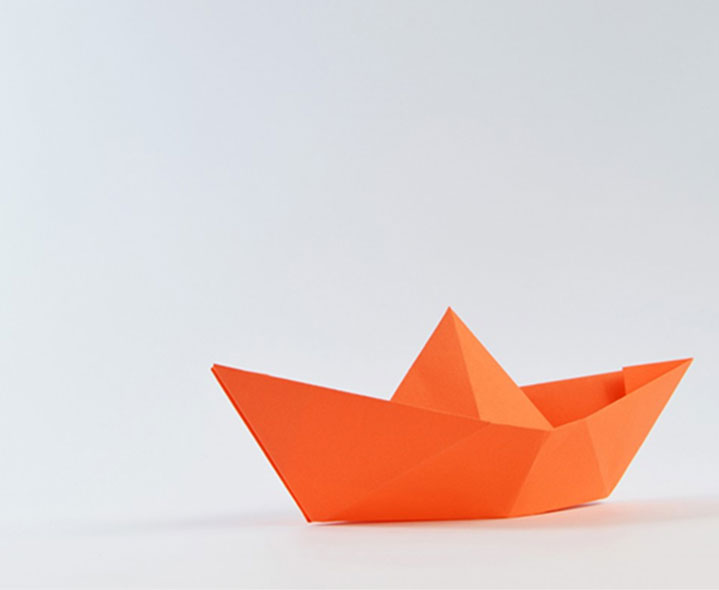
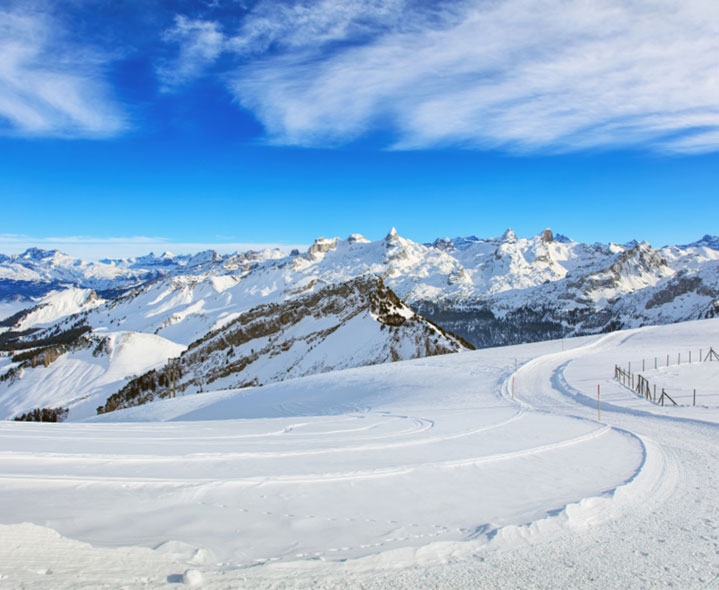